This is the second of two articles that asks a simple question: How can we produce new drugs faster, and at a lower cost? Can technology solutions help build a better mousetrap?
Our previous piece looked at mass-producing CAR-T therapies. Now we want to discuss how recent advances in artificial intelligence and machine learning (AI/ML) have opened up new opportunities that could help curb some of the costs of new drug development, while also significantly speeding-up the process.1
An especially timely example of how AI can work is the new COVID-19 Open Research Dataset (CORD-19).2 CORD-19 is a collaboration of private and government resources that contains over 130,000 scientific articles relating to the virus. Clearly, no one person can assimilate them all. This is a perfect application for AI/ML. Given enough data, AI/ML algorithms can identify patterns, make predictions, or classify new data much faster than any human.3
The hope is that the CORD-19 database will help researchers quickly understand what is known, and what questions still need to be answered about the disease.2
Setting expectations, defining terms
Just looking at headlines one might think that we will have AI/ML-designed drugs very soon – even now. One from September, 2019 reads, “Deep Genomics Advances What It Calls First AI Discovered Therapeutic Candidate.”4
Critics point out that what the company actually accomplished was to train its search tools to target very specific disease-causing splicing gene-problems. While that’s not an inconsequential feat, there are already drugs that work this way (for example, Spinraza®), that were discovered using conventional means. And, there are many additional steps to be completed before any eventual FDA-approved drug – none of which will be done by an AI.5
We will use the terms “AI” and “machine learning” in this paper, so let’s make sure we know why.
Artificial Intelligence (AI) can mean many things. The most extreme form of AI aims to simulate human intelligence – even consciousness.6 This kind of AI doesn’t exist, and may never exist.
But AI is also used as an umbrella term, with many subsets, such as Machine Learning (ML). According to a study by the General Accounting Office (GAO), Machine Learning is the form of AI that is currently being used in drug development.6
ML tools use large volumes of data to look at patterns and trends, and draw conclusions. ML systems can also automatically figure out how to improve their own results over time.7
Pharmaceutical makers are primarily using ML in the early steps of drug development, including drug discovery and preclinical research. Also, drug makers are beginning to use AI/ML to improve the clinical trial process.6
These applications are important because pharmaceutical manufacturers routinely cite the high cost of drug development as a major reason for high drug prices.8 The graphic below reports a 15 year timeline, with an overall cost of around $2 billion per new drug. But it also adds a crucial breakdown of costs by category.9 It illustrates how, when combined, the discovery, preclinical and clinical trial categories represent fully 80% of the drug development budget:
[Citation: Trends in Pharmacological Sciences. Artificial Intelligence for Clinical Trial Design. Published August 2019.]
Drug discovery
Let’s review some of the ways AI and ML are being used in the discovery, preclinical and clinical trial categories of new drug development.
Modern drugs are routinely pushing up against the boundaries of what is possible in the realm of science and technology. They have to, if only because the drugs that were the easiest to find have already been found. Now we have the much harder job of hunting for drugs to treat more complex and elusive conditions.1 (Learn more about how new drugs are developed.)
In virtual screening AI/ML tools are taught to use scientific rules to screen compounds for their effect on a selected target such as a protein, cell or organism.6 One company used ML to screen 12 million commercially available compounds for activity on a protein associated with heart failure. They purchased 200 compounds based on the results of that screen, and found that one-third of those were active. This is a far higher success rate than a conventional laboratory screen of a massive chemical library, which can be as low as 0.01%.6
AI/ML tools are also helping researchers filter known compounds for new therapeutic applications, and targeting already-developed drugs that may be candidates for repurposing.11 In one example, a new study reports that researchers from MIT have used AI to discover a new molecule that has been shown to effectively treat a common type of drug-resistant bacteria in mice.12
One of the unique contributions made by AI/ML here is that the tool uncovered it in a drug-repurposing database, where it had initially been tagged as a possible treatment for diabetes.13
In other words, even though this candidate drug was found in a completely unexpected place, AI gives researchers greater confidence that it would be worth pursuing through the more expensive development phases. As the co-author of the study remarked in an interview, “I do think this platform will very directly reduce the cost involved in the discovery phase of antibiotic development.”13
The GAO interviewed pharmaceutical manufacturers about using AI during drug discovery. One company calculates that by finding better drugs faster they can speed-up the entire process, to save $300 million to $400 million in R&D costs per successful new drug.6
Preclinical research
Traditional pretrial work relies on tools like chemical assays and mouse studies to test for liver toxicity. But even after an average of five years of testing, approximately 1/3 of new drugs fail in human trials for toxicity. The result is millions of dollars and years of effort wasted.1
So researchers trained an ML tool to model the molecular properties of FDA-approved drugs and drugs that failed for toxicity in clinical trials. The ML model was able to use what it ‘learned’ to predict whether a drug would fail for toxicity more accurately than some existing methods.6
Clinical trial phases
Approximately half of the total cost of drug development comes during the three trial phases, with 60% of that amount attributed the most complex Phase III trials. Based on the estimates given above, that means each failed clinical trial costs between $800 million and $1.4 billion.9
Every clinical trial has unique requirements for participating patients. One key cause for failed clinical trials is failure to recruit the best-suited patients – 32% of phase 3 trials fail because of patient recruitment and dropout problems.9 More dropouts require additional recruiting efforts to protect the statistical power of the results, which lead to delays and higher trial costs. AI/ML sorting can help match patients to trials more precisely and reduce the patient dropout rate.9
A second key cause for failed clinical trials is the lack of reliable adherence monitoring. Adherence monitoring often requires patients to routinely visit specified clinical locations, and to keep detailed records of their medication intake and a variety of other data. This can be an overwhelming task for patients, leading to on average, 40% of patients becoming non-adherent after 150 days in a clinical trial.9
Cloud-enabled AI/ML techniques, in combination with wearable sensors and video monitoring can be used to automatically and continuously collect patient data more reliably and efficiently than self-monitoring. That could mean improved adherence control, more reliable data outcomes, and potentially lower trial costs.9
The real role of AI/ML
Hopefully what has been clear is that the work AI/ML can do – while quite real and valuable – is not the glamorous stuff of science fiction. Sentient robots will not be inventing new drugs any time soon.
In truth, these are fairly utilitarian tools. They are doing what we have asked powered machinery to do since the industrial revolution: replace human effort on hard, repetitive, precision tasks – tirelessly, and very fast.13 In the 1700’s we designed looms that could pluck tiny cotton fibers, spin them into thread, and then weave thousands of threads into coats and blankets. Today, we program algorithms to sift and sort billions of bits of data, and weave them into coherent patterns that make sense to human eyes and minds.
In all likelihood, humans will continue to dominate the drug development process. One researcher explains that the real goal of AI/ML is to automate as much of the process as possible, so the chemists can focus on much higher level, and more difficult problems, like strategy.3
How much can AI save?
The biggest question is simply this: will increased efficiencies from AI/ML translate into lower drug costs? Certainly that is implied in many of the articles referenced throughout this discussion. However, in reality the field is so new, there is little hard data available regarding cost savings.1
On balance, considering the level of interest and investment in AI among drug companies, who are the most directly affected, it’s probably safe to say that they expect a considerable return.6, 14 But again, whether those savings will flow downstream to the end users is still unknown.
Related content
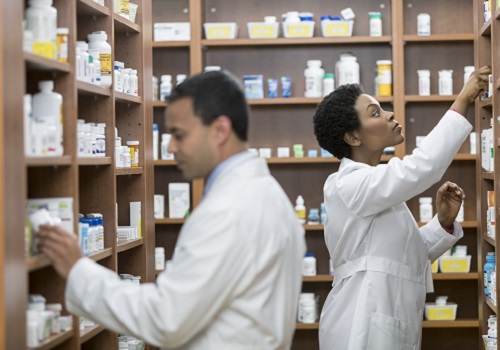
Rush to judgement?
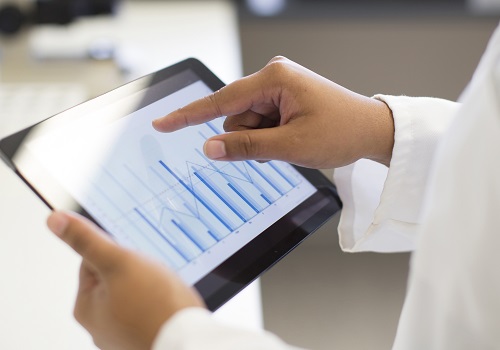
3 ways CRISPR will change the drug world
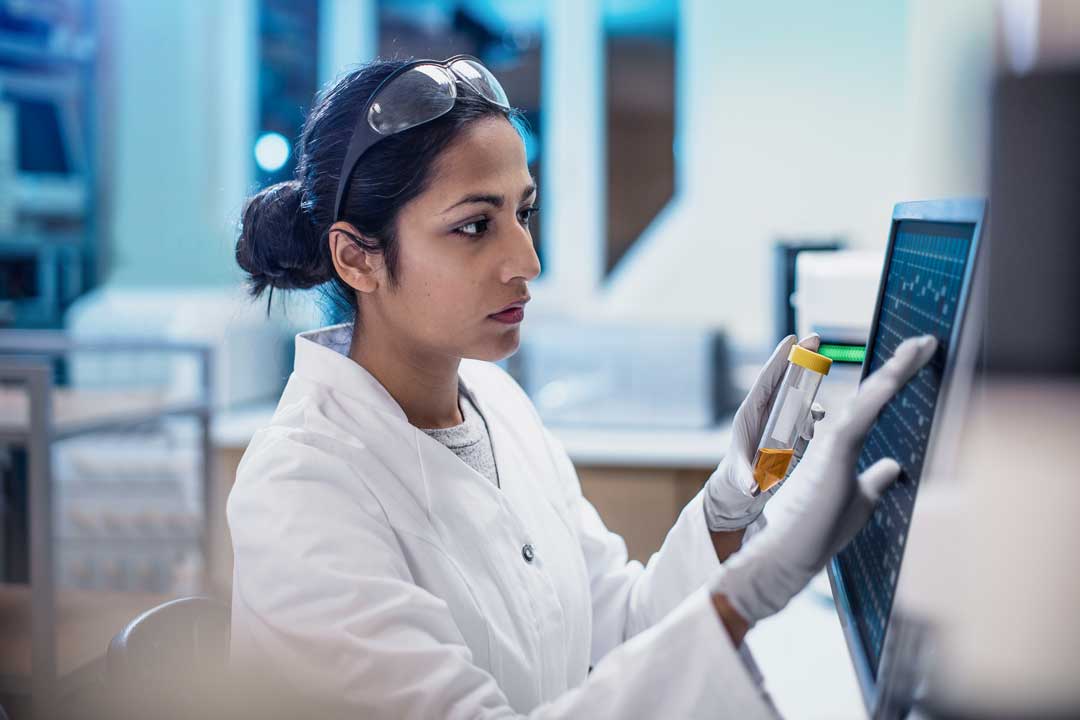
Will these 4 new drugs re-shape the market?
Sources
- Nature. Hunting for New Drugs with AI. Published December 18, 2019. Accessed March 9, 2020.
- White House Office of Science and Technology Policy. Call to Action to the Tech Community on New Machine Readable COVID-19 Dataset. Issued on March 16, 2020. Accessed on March 17, 2020.
- The Scientist. Artificial Intelligence Shakes Up Drug Discovery. Published April 30, 2019. Accessed February 19, 2020.
- Global Genes. Deep Genomics Advances What It Calls First AI Discovered Therapeutic Candidate. Published September 25, 2019. Accessed March 9, 2020.
- Derek Lowe's blog from Science Translational Medicine. An AI-Generated Drug? Posted September 30, 2019. Accessed March 25, 2020.
- U.S. Government Accountability Office (GAO). Artificial Intelligence in Health Care: Benefits and Challenges of Machine Learning in Drug Development. Reissued with revisions on Jan. 31, 2020. Accessed February 2, 2020.
- Scientific American. AI Doesn’t Actually Exist Yet: Many businesses claim they’re using it, but they’re kidding themselves—and they’re kidding you, too. Published November 12, 2019. Accessed March 25, 2020.
- Information Technology & Innovation Foundation. The Link Between Drug Prices and Research on the Next Generation of Cures. Published September 9, 2019. Accessed January 28, 2020.
- Trends in Pharmacological Sciences. Artificial Intelligence for Clinical Trial Design. Published August 2019. Accessed March 25, 2020.
- Nature. How artificial intelligence is changing drug discovery. Published May 30, 2018
- Future Drug Discovery. Artificial intelligence in drug discovery: what is new, and what is next? Published Online October 14, 2019. Accessed March 25, 2020.
- DistilInfo GovHealth Advisory. Aided by machine learning, scientists find a novel antibiotic able to kill superbugs in mice. Published February 27, 2020. Accessed March 10, 2020.
- Landes, David S. The Wealth and Poverty of Nations: Why Some are so Rich and Some so Poor. Published 1998 by W.W. Norton & Co. New York, NY.
- Clinical Therapeutics. A Study on the Application and Use of Artificial Intelligence to Support Drug Development. Published June 24, 2019. Accessed February 18, 2020. Accessed March 25, 2020.
STATEMENT REGARDING FINANCIAL INFLUENCE:
This article is directed solely to its intended audience about important developments affecting the pharmacy benefits business. It is not intended to promote the use of any drug mentioned in the article and neither the author nor Optum Rx has accepted any form of compensation for the preparation or distribution of this article.